OpenAI's Orion: Hitting the Data Wall and the Future of AI Model Development
Meta Description: OpenAI's Orion model, data limitations, AI scaling laws, synthetic data, future of AI development, challenges in AI model training, OpenAI's "Foundation" team.
Whoa, Nelly! The AI world is buzzing, and it's not all sunshine and rainbows. OpenAI, the undisputed heavyweight champion of AI, is facing a major hurdle: a shortage of high-quality data. This isn't just a minor setback; it's a potential game-changer that could significantly slow down the breakneck speed of AI advancements we've all come to expect. This isn't some fly-by-night startup struggling with funding; this is OpenAI, the company behind ChatGPT and GPT-4, grappling with a fundamental challenge that could redefine the future of artificial intelligence. Think of it like this: building a skyscraper without enough bricks – you can only get so far. The implications are huge, affecting everything from the performance of future models to the very way we approach AI research. Prepare to dive deep into the fascinating world of AI scaling laws, the looming "data wall," and OpenAI's innovative (and slightly desperate) attempts to navigate this uncharted territory. We'll explore the implications of this data crunch, the potential solutions being explored, and what it all means for the future of AI. Buckle up, because this ride is going to be wild!
The Data Crunch: A New Scaling Law?
The scaling law, a cornerstone of AI development for years, posited that bigger is better. More data, more parameters, more compute power – all equaled a more powerful AI model. Simple, right? Well, not so fast. OpenAI's new model, Orion (a name rumored to drop the familiar GPT-X moniker, hinting at a paradigm shift), is reportedly more powerful than anything OpenAI has created, but its improvement over GPT-4 isn't as dramatic as the leap from GPT-3. This suggests that the easy wins – simply throwing more data at the problem – are drying up. We're hitting a wall – a data wall.
This isn't just an OpenAI problem. Many in the AI community are beginning to question the old scaling law. As Tian Yuan-dong, a researcher at Meta AI, aptly pointed out, the closer AI gets to human-level intelligence, the harder it becomes to find new, relevant data. Those "corner cases," the rare and unexpected scenarios, become increasingly difficult to capture, acting like tiny cracks in a seemingly solid foundation. It's like trying to teach a child every single word in the dictionary; eventually, you run out of time and the child's understanding remains incomplete.
Epoch AI, a non-profit research organization, even predicts a potential data drought between 2026 and 2032. Their research suggests that the rate of data growth will soon fail to keep pace with the ever-increasing demands of larger AI models. This isn't some distant sci-fi dystopia; it's a very real, and very near, possibility.
OpenAI's Response: Synthetic Data and the "Foundation" Team
Facing this unprecedented challenge, OpenAI isn't throwing in the towel. They've established a dedicated "Foundation" team tasked with finding new ways to improve AI models even with limited new data. Their strategy? Embrace the power of synthetic data. The plan is to use AI to generate training data, a clever workaround to the scarcity of real-world data. Think of it as creating a digital goldmine – a vast, virtually limitless supply of training material. However, this approach isn't without its own set of challenges. Ensuring the quality and accuracy of synthetic data is crucial to prevent the model from learning inaccuracies or biases.
This isn't the first time OpenAI has grappled with data limitations. Rumors surfaced of GPT-5's training incorporating transcripts from YouTube videos to supplement the dwindling supply of high-quality text data. This highlights the creative, and sometimes desperate, measures needed to keep the AI innovation train chugging along.
The use of AI-generated data in Orion's training also raises concerns about the potential for the model to inherit the biases and limitations of the models used to generate its training data. This is a significant challenge that the "Foundation" team will need to address. It's a bit like teaching a child using only textbooks written by a biased author; the child may absorb those biases unknowingly.
The Human Element: Lilian Weng's Departure
Adding another layer of intrigue to this already complex picture is the departure of Lilian Weng, OpenAI's head of safety systems. While the reasons for her departure haven't been explicitly stated, it's hard not to wonder if the increasing pressure and challenges surrounding data limitations played a role. Her departure serves as a reminder that the human element – the brilliant minds behind the code – is just as crucial as the data itself.
Addressing the Challenges: A Look Ahead
The challenges facing OpenAI are significant, but the company's response demonstrates a commitment to innovation and problem-solving. This isn't just about building bigger models; it's about finding fundamentally new ways to approach AI development. The implications are far-reaching, affecting not only OpenAI but the entire AI field. We could be on the cusp of a new era in AI, one where the focus shifts from simply scaling up to creating more efficient and robust models.
Frequently Asked Questions (FAQs)
Here are some questions you might have about OpenAI's challenges and their potential solutions:
Q1: What is the "data wall" and why is it a problem for AI development?
A1: The "data wall" refers to the impending shortage of high-quality data needed to train increasingly large AI models. As models become more sophisticated, the need for diverse and nuanced data increases exponentially, outpacing the rate at which new data is being created. This limits the potential for further improvements in model performance.
Q2: How is OpenAI trying to overcome the data limitations?
A2: OpenAI is exploring the use of synthetic data – data generated by AI – to supplement the dwindling supply of real-world data. They've also created a "Foundation" team to explore innovative approaches to model training.
Q3: What are the potential risks of using synthetic data for training AI models?
A3: Synthetic data, if not carefully generated and vetted, can introduce biases and inaccuracies into the model. It's crucial to ensure that the synthetic data accurately reflects the real-world distribution of data to prevent unintended consequences.
Q4: What is the significance of Lilian Weng's departure from OpenAI?
A4: While the specific reasons haven't been publicly stated, her departure highlights the human element in AI development and the pressures faced by those working on the cutting edge of AI research.
Q5: Will the scaling law still hold true in the future?
A5: The traditional scaling law, which emphasized the importance of sheer scale, may need revision. The current challenges suggest a need for more sophisticated approaches that prioritize data quality and efficiency over simply increasing size.
Q6: What does this mean for the future of AI?
A6: The current challenges may lead to a paradigm shift in AI development. We may see a greater focus on data augmentation techniques, improved model architectures, and more efficient training methods. The focus will likely shift from just "bigger" to "better" and "smarter."
Conclusion
OpenAI's encounter with the "data wall" is a pivotal moment in the history of AI. It's a wake-up call, highlighting the limitations of the current approach to AI development and forcing the field to re-evaluate its strategies. While challenges remain, OpenAI's innovative response, along with the broader industry's response to this hurdle, promises a future where AI development is not only more efficient but also more responsible and ethical. The race is on—not just to build the biggest models, but to build the best models, and this data crunch might just be the catalyst we need to achieve that. The journey continues, and the future of AI is far from written.
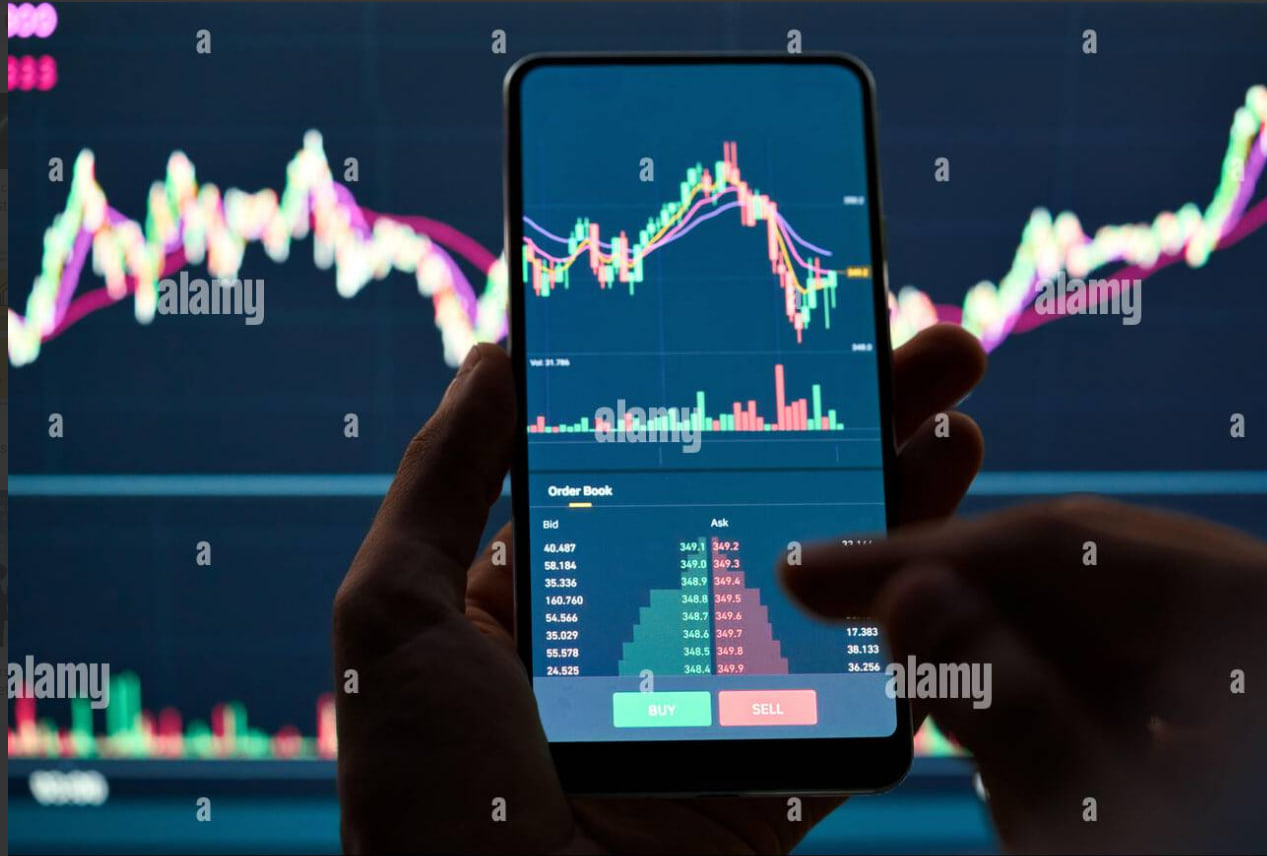